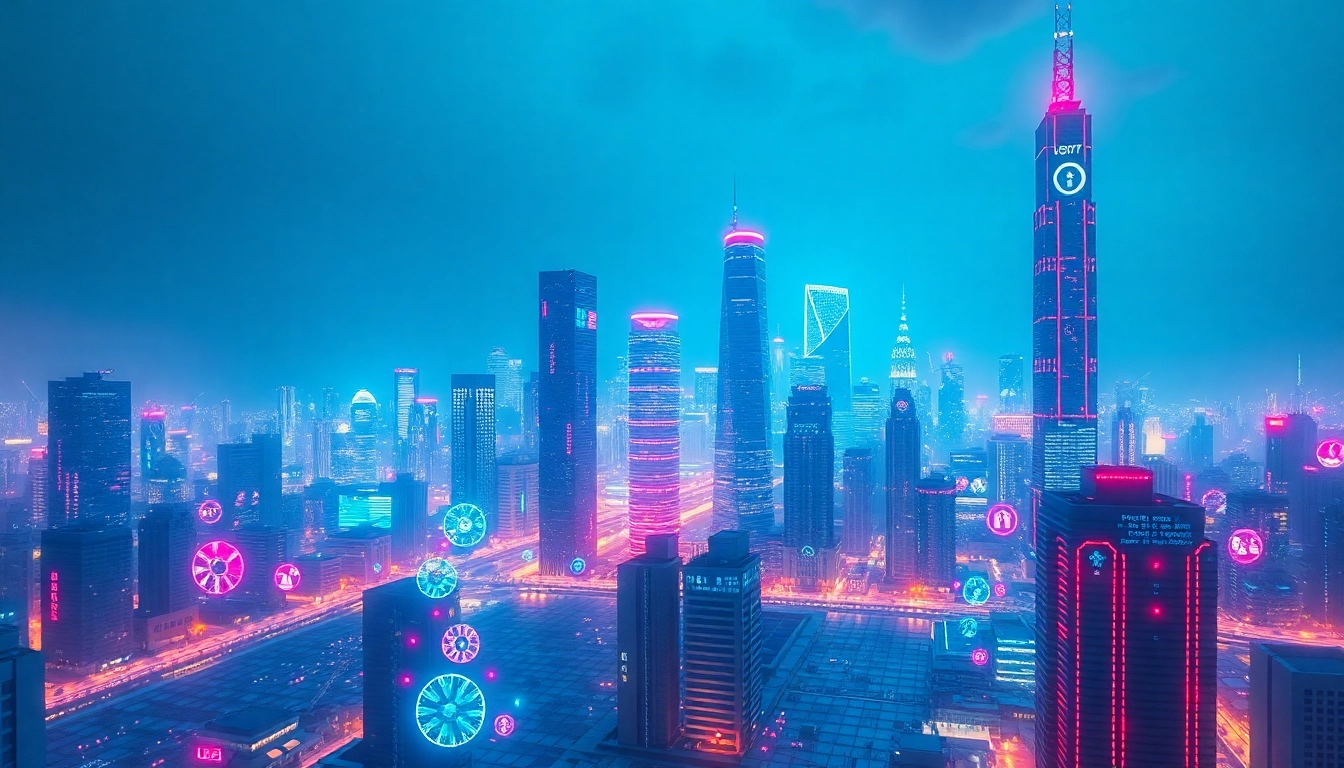
Understanding Agentic AI
As artificial intelligence (AI) continues to transform industries and redefine the boundaries of technology, understanding its various forms becomes essential. One of the most intriguing concepts in the AI landscape is Agentic AI, a term used to describe AI systems that operate independently to make decisions and take actions without ongoing human input. This article delves into the definition of agentic AI, its key characteristics, and how it differs from other AI paradigms like generative AI.
Definition of Agentic AI
Agentic AI refers to sophisticated systems that are capable of autonomous operation. These systems can analyze their environments, identify problems, and implement solutions without needing constant supervision from humans. The essence of agentic AI lies in its ability to set goals, devise strategies to achieve those goals, and execute actions based on contextual data and prior learning. By leveraging machine learning, knowledge representation, and reasoning, agentic AI can adapt over time, enhancing its decision-making capabilities in real-world applications.
Key Characteristics of Autonomous Systems
When exploring agentic AI, several critical features can be identified:
- Autonomy: The hallmark of agentic AI is its ability to operate without human intervention. Once deployed, these systems make decisions independently, responding to changes in their environment.
- Decision-making capabilities: Agentic AI systems utilize complex algorithms to evaluate scenarios and determine the best course of action among multiple alternatives.
- Learning and adaptation: Through continuous interaction with their environment, agentic AI systems learn from outcomes and refine their strategies, improving their performance over time.
- Goal-oriented behavior: Unlike traditional AI, which might only perform specific tasks, agentic AI focuses on accomplishing broader objectives, ensuring its actions align with pre-defined goals.
Differences Between Agentic AI and Generative AI
To appreciate the nuances of agentic AI, it is helpful to contrast it with generative AI. While both belong to the broader AI category, their functionalities diverge significantly:
- Goal Orientation: Agentic AI is designed to achieve predefined objectives through autonomous decision-making. In contrast, generative AI is primarily focused on content creation, generating new data based on patterns and inputs provided by users.
- Decision vs. Creation: Generative AI creates outputs such as text, images, or music, often requiring prompts from users. In comparison, agentic AI actively decides and executes actions based on its assessment of situations.
- Interactivity: Agentic AI operates continuously and adjusts its approaches based on environmental feedback, whereas generative AI tends to be more static until prompted to produce new content.
Applications of Agentic AI
The versatility of agentic AI allows for its application across various fields, enhancing efficiency and effectiveness. Below, we explore its real-world applications and the industries that are increasingly adopting these solutions.
Real-World Examples
Numerous examples showcase the capabilities of agentic AI:
- Supply Chain Management: Agentic AI can optimize logistics by predicting demand patterns, improving inventory management, and reducing waste through autonomous decision-making.
- Healthcare: In medical settings, agentic AI systems assist in diagnostics and patient management by analyzing patient data and suggesting appropriate treatment plans.
- Finance: Financial institutions utilize agentic AI for risk assessment, fraud detection, and algorithmic trading, allowing for rapid and informed decision-making.
- Autonomous Vehicles: These systems exemplify agentic AI; they process data from their surroundings, make real-time driving decisions, and learn from diverse driving conditions.
Industries Adopting Agentic AI
Agentic AI’s capability to operate autonomously has found favor in several industries:
- Retail: Retailers use agentic AI for personalized marketing, autonomous inventory restocking, and customer service chatbots that handle inquiries without human oversight.
- Cybersecurity: Agentic AI systems can autonomously identify and neutralize threats in real-time, significantly enhancing an organization’s security posture.
- Manufacturing: Smart factories employ agentic AI for quality control, predictive maintenance, and supply chain optimization, streamlining operations while reducing costs.
- Telecommunications: AI offers proactive troubleshooting and maintenance for telecommunications networks, ensuring uninterrupted communication services.
Benefits of Implementing Agentic AI Solutions
Embracing agentic AI brings a plethora of advantages:
- Increased Efficiency: By automating routine tasks, agentic AI allows employees to focus on higher-level functions, fostering productivity.
- Enhanced Decision-Making: With real-time data processing and autonomous strategy formulation, organizations can make faster and more accurate decisions.
- Cost Reduction: Businesses can reduce operational costs by minimizing the need for extensive human oversight and intervention in various corporate functions.
- Scalability: Agentic AI can accommodate increased workloads without the need for proportional increases in human resources, making scaling more manageable.
Challenges in Adopting Agentic AI
Despite its promising potential, the adoption of agentic AI is not without challenges. Organizations must navigate several hurdles to successfully implement these technologies.
Technical Barriers and Limitations
One of the foremost challenges in deploying agentic AI involves technical barriers, including:
- Data Quality: The effectiveness of agentic AI relies on access to large volumes of high-quality, relevant data. Ensuring the integrity and cleanliness of this data can be a considerable undertaking.
- Integration with Existing Systems: Many organizations grapple with integrating new AI systems into their existing technology frameworks. Legacy systems may lack compatibility with agentic AI technologies, causing disruptions.
- Complexity of Development: Designing and developing agentic AI systems involves significant expertise in machine learning, natural language processing, and robotics, which may be beyond the reach of many organizations.
Ethical Considerations and Regulations
As agentic AI systems take on more autonomous roles, ethical considerations rise to the forefront:
- Accountability: Questions arise about who is responsible for the decisions made by autonomous systems. Establishing a clear accountability framework becomes crucial.
- Bias and Fairness: AI systems can perpetuate or even exacerbate existing biases present in training data. Ensuring fairness and neutrality in decision-making processes is an ongoing challenge.
- Privacy: The collection and utilization of data by agentic AI systems pose significant privacy concerns, emphasizing the need for robust data governance and compliance with regulations.
Addressing Public Concerns
Given the transformative potential of agentic AI, public apprehension concerning job displacement and loss of control is prevalent. To mitigate these concerns, stakeholders must actively engage in:
- Education: Raising awareness about how agentic AI functions and its benefits can help alleviate fears surrounding AI’s role in society and the workplace.
- Transparency: Businesses should be transparent about how AI technologies are employed, enabling individuals to see their benefits and fostering trust in AI systems.
- Workforce Re-training: Proactively reskilling the workforce prepares employees for roles that complement AI technology, ensuring that human oversight remains integrated into AI processes.
The Future of Work with Agentic AI
As agentic AI continues to evolve, its influence on the workforce will grow. Predicting how it will reshape job roles and responsibilities is crucial for preparing for an increasingly automated future.
Predictions for Workforce Integration
As industries progress towards automation, it is anticipated that agentic AI will lead to:
- Creation of New Job Categories: The emergence of specialized roles focused on managing, interpreting, and providing oversight for AI systems is expected.
- Enhanced Collaboration: AI will increasingly work alongside humans in various capacities, requiring individuals to adapt and learn to harness AI tools effectively.
- Increased Emphasis on Soft Skills: As technical tasks become automated, skills such as creativity, emotional intelligence, and critical thinking will gain prominence.
Impact on Job Roles and Responsibilities
The evolving landscape wrought by agentic AI will significantly alter job roles:
- Shift from Routine to Strategic Work: Employees across sectors will find themselves engaged in more strategic and less repetitive tasks, enhancing job satisfaction.
- AI Ethics and Governance Roles: Businesses will likely cultivate positions dedicated to overseeing the ethical implications of AI and ensuring compliance with evolving regulations.
- Team-based Structures: The rise of AI may lead to restructuring of teams, where humans and AI systems collaborate seamlessly to achieve organizational objectives.
Upskilling for an AI-Driven Economy
To thrive in a world dominated by agentic AI, workforce upskilling is paramount. Organizations can implement the following strategies:
- Investing in Training Programs: Targeted training that enhances both technical and soft skills will prepare employees for new roles within an AI-driven economy.
- Emphasizing Lifelong Learning: Instilling a culture of continuous learning ensures employees remain adaptable and equipped to handle technological change.
- Collaboration with Educational Institutions: Partnerships with universities and training programs can facilitate the delivery of relevant skills before employees enter the workforce.
How to Get Started with Agentic AI
Organizations looking to harness the power of agentic AI must approach implementation strategically. Here are essential steps to guide the process.
Steps for Implementation
To effectively implement agentic AI solutions, consider the following steps:
- Define Objectives: Clearly outline the specific goals you aim to achieve with agentic AI implementation. Understanding the desired outcomes will guide your approach.
- Assess Data Needs: Identify the types of data required for training AI models and ensure mechanisms are in place to collect high-quality data.
- Choose the Right Technology: Evaluate various agentic AI platforms that align with your organization’s needs and capabilities.
- Build Cross-Functional Teams: Gather experts from various disciplines, including AI specialists, domain experts, and IT staff, to collaborate on the implementation strategy.
- Pilot Test: Before full-scale deployment, conduct pilot tests in controlled environments to understand how agentic AI solutions perform and make necessary adjustments.
Measuring Success and Performance Indicators
Measuring the success of agentic AI initiatives is crucial to determine their effectiveness. Key performance indicators (KPIs) may include:
- Efficiency Gains: Evaluate improvements in productivity resulting from AI-driven automation processes.
- Cost Savings: Assess reductions in operational costs due to the implementation of agentic AI systems.
- Quality of Decision-Making: Examine the impact of AI on decision-related outcomes to ensure that the system is meeting business objectives.
Resources for Further Learning
As interest in agentic AI continues to grow, numerous resources are available for those looking to deepen their understanding, including:
- Online Courses: Platforms such as Coursera and edX offer courses on AI and machine learning tailored to different experience levels.
- Books: Titles focused on AI ethics, decision-making, and automation provide valuable insights into the implications of agentic AI technology.
- Industry Conferences: Attending conferences and workshops can connect professionals with experts and provide networking opportunities in the AI field.