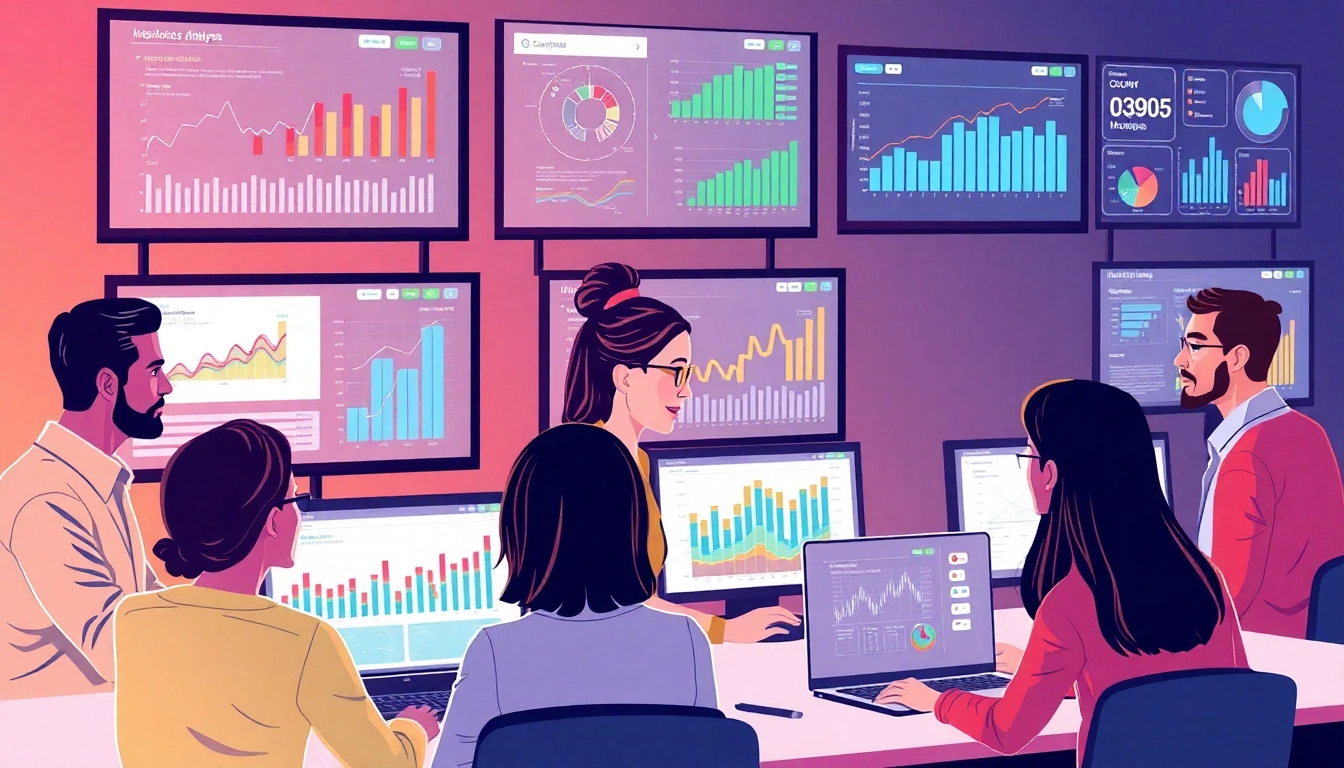
Understanding AI Sentiment Analysis
In the digital age, where the amount of textual data generated daily is staggering, understanding the emotions expressed in this data has become exceptionally important for businesses and individuals alike. AI sentiment analysis refers to the use of artificial intelligence technologies to interpret and classify emotions embedded in text data. This capability not only fosters enhanced customer engagement but also provides businesses with actionable insights for strategic decision-making.
What is Sentiment Analysis?
Sentiment analysis is a computational technique used to analyze textual data and determine the emotional tone behind the text. Often used in marketing, customer service, and social media analysis, sentiment analysis categorizes sentiments into positive, negative, or neutral classifications. This can involve analyzing diverse content types, including social media posts, product reviews, and feedback surveys.
Importance of Emotion in Data Interpretation
Emotions play a crucial role in how data is interpreted and applied. Understanding customer sentiment can help organizations gauge public perception, manage reputation, and shape product development. For example, businesses can proactively adjust their marketing strategies based on public feedback, thereby achieving a better alignment with customer preferences.
Key Features of AI Sentiment Analysis Tools
AI sentiment analysis tools typically come equipped with several key features that enhance their functionality:
- Natural Language Processing (NLP): NLP techniques enable the interpretation of human languages, allowing machines to process and analyze text in a meaningful way.
- Advanced Machine Learning Models: Some tools utilize deep learning models that iteratively learn from vast amounts of data, improving their accuracy over time.
- Real-Time Analysis: Many AI sentiment analysis tools provide real-time analytics, allowing businesses to monitor sentiment changes instantaneously.
- Multi-Language Support: As businesses operate globally, effective sentiment analysis tools must handle multiple languages and dialects.
Approaches to AI Sentiment Analysis
Natural Language Processing Techniques
Natural Language Processing is the backbone of sentiment analysis. It combines computational linguistics with machine learning algorithms to analyze and understand human language. Key techniques include:
- Tokenization: This involves breaking down text into smaller units (tokens) for analysis.
- Part-of-Speech Tagging: Identifying the grammatical parts of speech improves understanding of context.
- Named Entity Recognition (NER): This helps detect entities like names, organizations, and locations in the text and categorize them accordingly.
Machine Learning Algorithms in Sentiment Analysis
Machine learning plays a vital role in identifying patterns in sentiment analysis. Algorithms such as logistic regression, support vector machines, and random forests are commonly used for this purpose. Each of these algorithms has its strengths and is chosen based on the data characteristics and requirements of the project.
Deep Learning vs. Traditional Methods
Deep learning approaches, particularly those leveraging neural networks, have seen growing popularity in sentiment analysis due to their ability to manage vast datasets and unearth complex non-linear relationships in the data. While traditional methods are often easier to implement and explain, they may overlook nuances that deep learning can capture efficiently.
Applications of AI Sentiment Analysis
Enhancing Customer Experience
By analyzing customer interactions and feedback, businesses can utilize sentiment analysis to enhance customer experience. For instance, a company could analyze feedback from a recent product launch to identify sentiments around new features or services, allowing them to make data-driven adjustments to their offerings.
Market Trend Analysis and Predictions
Sentiment analysis can also be used to study market trends and consumer behavior. By monitoring social media and other platforms for mentions of specific products or brands, businesses can gain insights into public perception which will help forecast demand and adjust marketing strategies accordingly.
Reputation Management for Brands
In today’s fast-paced social media landscape, managing brand reputation is crucial. AI sentiment analysis tools enable companies to monitor mentions and comments made about their brand in real-time, allowing them to respond swiftly to negative sentiment while also leveraging positive interactions.
Challenges in AI Sentiment Analysis
Data Quality and Accuracy Issues
The accuracy of sentiment analysis largely depends on the quality of the data being analyzed. Low-quality data can lead to misleading outcomes. Ensuring high-quality data requires rigorous data cleaning and preprocessing techniques to handle issues like noise and irrelevant information.
Understanding Context and Nuances
Human language is complex, filled with idioms, sarcasm, and non-literal expressions. AI sentiment analysis models often struggle with these nuances, leading to misinterpretations. Continuous training and model refinement are necessary to enhance their contextual understanding.
Integration with Existing Systems
Implementing AI sentiment analysis solutions often requires integration with existing IT systems, which can present operational and technical challenges. Organizations must ensure that their sentiment analysis tools seamlessly connect with their data sources and business processes for optimal effectiveness.
Future Trends in AI Sentiment Analysis
Role of Generative AI in Advanced Analysis
Generative AI models are poised to enhance sentiment analysis by improving contextual understanding and the extraction of sentiment nuances. These models can synthesize new data based on learned patterns, enabling more accurate sentiment classification.
Personalization and Predictive Analytics
The future of sentiment analysis will likely be marked by increased personalization. Businesses will utilize sentiment data not just to react to customer feedback, but to anticipate needs and tailor experiences that resonate on an individual level.
Ethical Considerations and Data Privacy
As AI sentiment analysis tools evolve, ethical considerations will become paramount. Businesses must navigate the delicate balance between leveraging consumer data to optimize engagement and respecting individual privacy rights. Transparency and clarity in data usage policies will be essential.