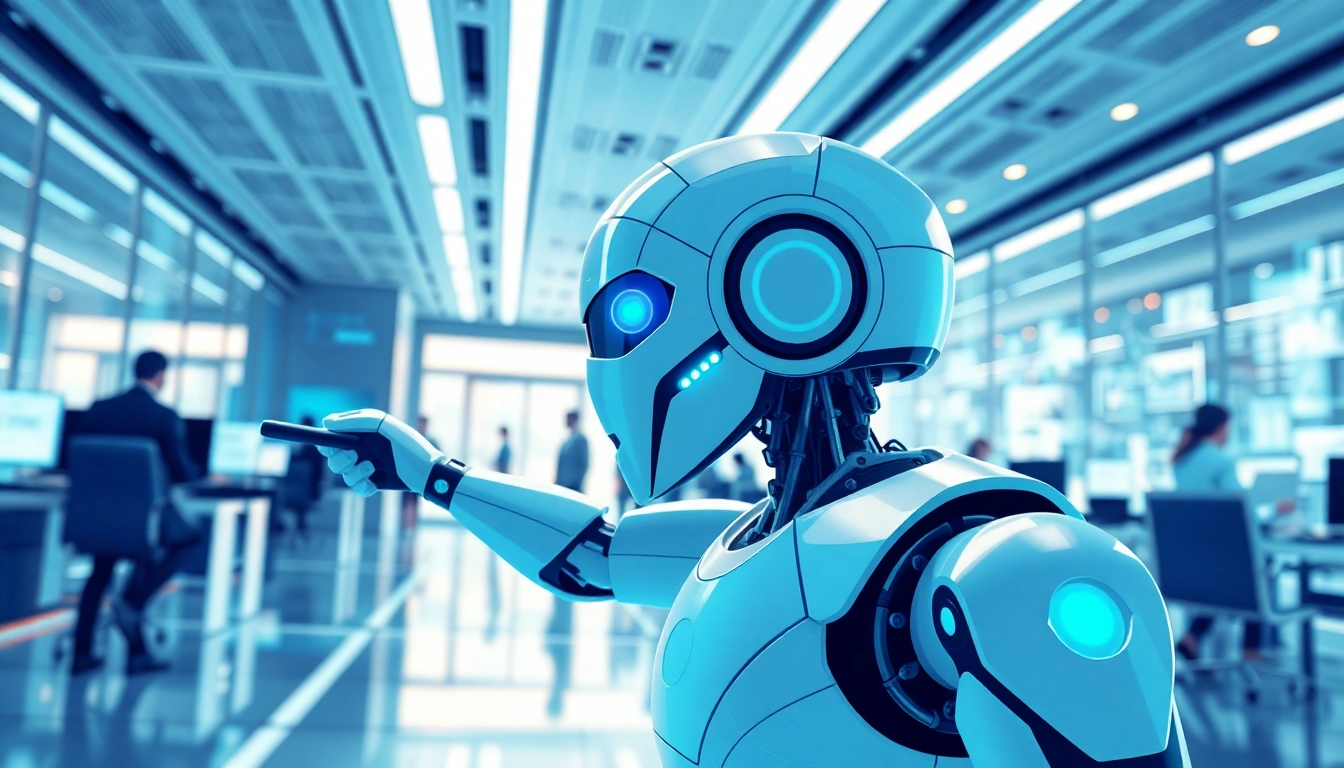
1. Introduction to Agentic Human AI
In an era where artificial intelligence (AI) is increasingly becoming a cornerstone of technological advancement, understanding concepts like Agentic Human AI is crucial. Agentic Human AI refers to AI systems that possess the capability to act autonomously toward achieving specific goals without requiring continuous human input. This article explores the breadth of Agentic Human AI, delving into its characteristics, historical evolution, practical applications, ethical considerations, and implications for the future of work.
What is Agentic Human AI?
Agentic Human AI encompasses systems that leverage machine learning and data analytics to function with a degree of autonomy. These systems can perceive their environment, make decisions based on that data, and take action independently. Unlike traditional AI, which often relies on explicit guidance from human operators for every task, Agentic AI can manage complexities and adapt to new situations autonomously. Such systems are particularly valuable in dynamic settings where rapid decision-making is vital.
Key Characteristics and Features
Agentic Human AI systems exhibit several key characteristics that distinguish them from conventional AI models:
- Autonomy: The primary feature of agentic AI is its ability to act independently, making decisions without human intervention.
- Adaptability: These AIs can adjust their actions based on changes in their environment, learning from new data to optimize their performance continuously.
- Goal-Oriented Behavior: Agentic AI functions are designed with specific objectives in mind, enabling them to focus on achieving defined outcomes effectively.
- Multi-Step Problem Solving: Many agentic AIs can handle complex, multi-step problems that require extensive reasoning and planning.
The Importance of Autonomy in AI
The drive for autonomy in AI stems from the need for efficiency and scalability in operations. Autonomy allows AI systems to function effectively away from human oversight, thereby increasing productivity and enabling companies to scale their operations without proportionate increases in human labor. Important implications include reduced operational costs, decreased response times, and the ability to undertake tasks that may be dangerous for humans.
2. The Evolution of AI Agents
Historical Context of Autonomous Systems
The development of autonomous systems can be traced back to the early days of artificial intelligence research in the 1950s and 1960s. Initially, AI was focused mostly on narrow tasks, such as simple rule-based decision-making and pattern recognition. As algorithms and computational power advanced, researchers began exploring more sophisticated types of autonomy, leading to the emergence of machine learning and deep learning techniques.
Milestones in Agentic AI Development
The evolution of agentic AI has reached several significant milestones:
- Expert Systems (1980s): Early AI systems that utilized rule-based logic to solve specific problems, laying the groundwork for future developments in autonomous systems.
- Machine Learning (1990s): The introduction of algorithms that allowed systems to learn from data without explicit programming.
- Deep Learning (2010s): Advanced neural networks that enabled AI to process vast amounts of data at unprecedented speed, enhancing its capability for independence.
- Reinforcement Learning (2010s): Techniques that allow AI to learn optimal actions through trial and error, further enhancing autonomy.
Comparing Agentic, Narrow, and General AI
Understanding the differences between agentic AI and other forms of AI is essential:
- Narrow AI: These systems are designed for specific tasks and cannot operate outside their programming. They rely heavily on human guidance and predefined rules.
- Agentic AI: Can perform complex tasks autonomously and make decisions based on real-time data, serving as a bridge between narrow AI and general AI.
- General AI: A theoretical AI that would be able to understand, learn, and apply intelligence across a broad range of tasks, similar to human cognitive abilities.
3. Practical Applications of Agentic Human AI
Use Cases in Various Industries
Agentic Human AI has found applications in numerous sectors, demonstrating its versatility and effectiveness:
- Healthcare: AI systems that help diagnose diseases by analyzing patient data and medical images autonomously, leading to quicker, more accurate diagnoses.
- Finance: Autonomous trading systems that analyze market data and make investment decisions without human intervention.
- Manufacturing: AI robots that optimize production lines by making real-time adjustments to improve efficiency and reduce waste.
- Retail: AI customer service agents that provide personalized shopping experiences and resolve customer inquiries independently.
- Transportation: Self-driving vehicles that navigate and make driving decisions without human input, showcasing one of the most advanced applications of agentic AI.
Benefits of Implementing Autonomous AI
The implementation of Agentic Human AI can lead to significant benefits, including:
- Increased Efficiency: Automating routine tasks reduces the time and resources spent on operational processes.
- Enhanced Decision Making: Agentic AI can analyze data far more quickly and accurately than humans, leading to superior outcomes.
- Cost-Effectiveness: Reducing reliance on human labor can result in substantial savings for organizations.
- Improved Safety: Autonomous systems can take over dangerous tasks, reducing the risk of human injury or error.
Real-World Examples: Success Stories
Several companies have successfully integrated Agentic Human AI into their operations:
- IBM Watson: Used in healthcare to analyze medical data, assist in diagnostics, and support treatment plans.
- Amazon: Utilizes AI in its logistics and supply chain management, automating inventory control and order fulfillment processes.
- Waymo: A leader in self-driving technology, utilizing agentic AI to develop autonomous vehicles that navigate complex urban environments.
4. Ethical Considerations and Challenges
Addressing Concerns About AI Autonomy
As AI systems become more autonomous, ethical concerns arise regarding their decision-making capabilities. These concerns include biases in algorithms, transparency in AI decisions, and the implications of automating jobs.
Balancing Control and Independence
It is essential to find a balance between allowing AI systems to operate independently and maintaining human oversight. This balance ensures that while AI can act autonomously, it does so within a framework that secures human values and ethical principles.
Future Ethical Frameworks for Agentic AI
The development of ethical frameworks around Agentic Human AI is crucial. These frameworks should address data privacy concerns, accountability of AI actions, and guidelines for developing AI systems that align with societal norms.
5. The Future of Work with Agentic AI
Impact on Job Roles and Skills
The rise of Agentic AI is poised to alter the landscape of the workforce significantly. While some jobs may become obsolete, many new roles will emerge, particularly in AI oversight, maintenance, and ethics. Workers will need to develop new skills relevant to collaborating with autonomous systems.
How Agentic AI Will Shape Work Environments
We can expect to see work environments transforming into more collaborative spaces where human workers and AI systems work in tandem. This evolution could lead to increased creativity and innovation as humans focus more on strategic and complex problem-solving tasks, while the AI handles operational efficiencies.
Preparing for the Future: Skill Development Strategies
To prepare for this shift, organizations and educational institutions should emphasize training in AI literacy, data analysis, critical thinking, and interpersonal skills. Encouraging adaptability and continuous learning will empower workers to thrive in future work environments where Agentic AI is prevalent.