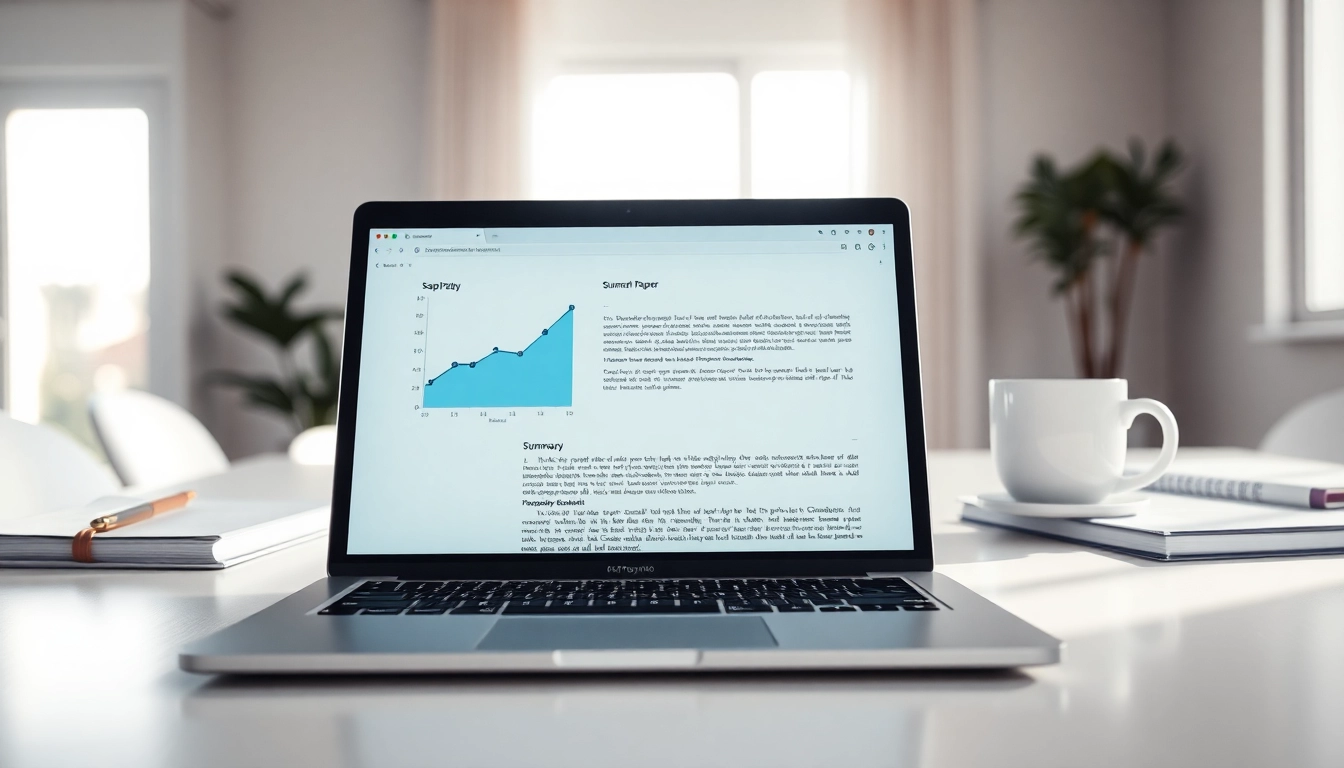
Understanding the Need for AI Summaries
In an age where information is abundant but time is limited, the need for effective summarization tools has never been more critical. Researchers, educators, and students are inundated with data – from complex scientific papers to analytical reports – all of which require significant time to digest and comprehend. As the volume of published research continues to grow exponentially each year, many have turned to AI for assistance. An ai summary of papers can provide a streamlined approach to grasping essential insights quickly and effectively.
Academic Challenges in Paper Summarization
The academic community faces myriad challenges when it comes to paper summarization. Researchers often struggle with cognitive overload, attempting to keep up with the latest findings while maintaining the quality of their original work. Key problems include:
- Volume of Information: With millions of papers published yearly, determining relevance can be daunting.
- Complex Language: Academic writing often uses jargon that can obscure meaning for those outside a specific field.
- Time Constraints: Busy schedules limit the ability to conduct thorough literature reviews.
- Data Overload: Extracting pertinent insights from extensive data sets can lead to analysis paralysis.
The Role of AI in Enhancing Learning
AI is transforming academic education and research methodologies. By leveraging sophisticated algorithms, AI can process vast amounts of data and generate coherent summaries that encapsulate key findings and implications. This technology has various applications, including:
- Accessibility: AI tools can simplify complex texts, making them more accessible to non-experts.
- Efficiency: Researchers can quickly glean crucial information without reading entire papers.
- Personalization: AI can tailor summaries based on user preferences, enhancing the learning experience.
Benefits of AI Summary of Papers
The integration of AI in summarizing academic papers offers numerous advantages:
- Time-saving: Users can save hours that would otherwise be spent reading lengthy documents.
- Improved Retention: Summaries allow for better retention of information, helping users focus on key points.
- Enhanced Research Capability: Using AI summarizes enables comprehensive literature reviews faster, leading to more informed research outcomes.
- Collaboration: AI tools facilitate better collaboration by providing unified insights that all team members can understand.
How AI Summarizes Papers: A Deep Dive
To grasp the significance of AI summaries, it is essential to understand how these systems operate. The underlying technologies are fascinating and complex.
Algorithms Behind AI Summarization
AI summarization tools employ various algorithms, predominantly natural language processing (NLP) and machine learning techniques:
- Extractive Summarization: This method pulls sentences directly from the text, prioritizing those that are deemed most important based on statistical measures.
- Abstractive Summarization: Unlike its extractive counterpart, abstractive summarization generates new sentences that capture the essence of the document, often paraphrasing original content.
- Deep Learning: Advanced models like transformers (including BERT and GPT) are being used to improve understanding of context and semantics for more relevant summarization.
Data-Driven Insights and Accuracy
Data-driven insights are critical in evaluating the effectiveness of AI summarization tools. Accuracy is often measured against human-generated summaries, and significant advancements have been made:
- Benchmark datasets have been created to train models, allowing for continuous refinement and enhancement.
- Feedback mechanisms enable AI systems to learn from user interactions, thus improving their precision over time.
- Evaluation metrics such as ROUGE and BLEU assess the quality and relevance of generated summaries.
User-friendly Tools and Features
Modern AI summarization tools are designed to be user-friendly, catering to a broad audience. Some of the notable features include:
- Customization: Many tools allow users to set preferences regarding length, style, and focus areas for summaries.
- Integration: Key platforms integrate seamlessly into existing research workflows and databases.
- Interactive Interfaces: User interfaces often support easy navigation and intuitive design, improving user experience.
Popular AI Summarization Tools Comparison
With several AI summarization tools available, understanding their unique specifications helps potential users select the tool that best fits their needs. Here’s a comparison of some leading platforms:
Overview of Leading Competitors
Several notable companies and tools stand out in the robust AI summarization market:
- SciSummary: Offers concise summaries of scientific articles, aiming to improve the efficiency of literature reviews.
- Paperguide: Delivers customizable summaries tailored to academic requirements.
- QuillBot: Known for its effective text summarization while maintaining user-friendly operations.
- Scholarcy: Allows users to create summaries for various academic formats, including articles and textbooks.
Key Features and Pricing Models
When choosing an AI summarization tool, consider the following:
- Pricing: Many platforms offer free trials, with scalable pricing for advanced features. Features may vary between free and premium subscriptions, impacting overall usability.
- Performance: Tools may differ in terms of summarization speed, accuracy, and ability to integrate advanced features like citation generation.
- Support: Customer support options help users troubleshoot and maximize their use of the tools.
User Feedback and Performance Metrics
User reviews often provide insight into the strengths and weaknesses of different platforms. Performance metrics reveal:
- Overall satisfaction ratings indicating the effectiveness of the summarization.
- Use case-specific performance evaluations, such as how well a tool performs with specific academic disciplines.
- Feedback on usability can help identify potential barriers or challenges for new users.
Integrating AI Summaries into Your Workflow
Adopting AI summary tools into research workflows can significantly enhance productivity. However, best practices must be implemented for maximum benefit.
Best Practices for Efficient Research
Utilizing AI summarization effectively requires specific methodologies:
- Define clear objectives regarding what information is necessary from the summaries.
- Incorporate summarization tools early in the research process to guide literature searches.
- Make use of feedback from AI summaries to understand gaps in knowledge and areas for further investigation.
Common User Pitfalls to Avoid
While AI tools offer immense advantages, certain pitfalls must be avoided:
- Over-reliance on summaries without engaging with the original content can lead to misunderstandings of complex topics.
- Failure to customize AI tools can result in irrelevant or overly simplified summaries that miss critical insights.
- Ignoring the feedback mechanisms available in these tools can stymie improvements in accuracy and relevance.
Enhancing Collaborative Learning with AI
Collaboration is critical in research settings. Utilizing AI summary tools can enhance group projects and discussions:
- Foster open dialogue about summarized content to enrich understanding across diverse academic backgrounds.
- Incorporate AI-generated summaries into team meetings to streamline discussions and allocate time efficiently.
- Encourage team members to raise questions and insights gained from AI summarization to promote deeper analysis.
Future Trends in AI Summarization Technology
The landscape for AI summarization is evolving rapidly. As technology progresses, new trends will emerge that significantly enhance research methodologies.
Predictions for Upcoming Developments
Future advancements may include:
- Improved models that deliver even higher accuracy rates in understanding context and semantics.
- Greater integration of AI summarization tools with other research platforms across different fields.
- Enhanced user experience features, making AI tools more accessible to those with diverse tech backgrounds.
The Evolving Landscape of Academic Research
As AI technology continues to evolve, the academic research landscape will witness transformative changes, such as:
- Increased collaboration between AI researchers and academic institutions to optimize research outputs.
- Emerging markets for AI summarization tools tailored toward specific academic disciplines.
- Shifts in how academic merit is assessed, potentially moving toward data-driven measures of research efficiency.
Strengthening Accessibility with AI Tools
AI summarization tools will play a critical role in increasing accessibility in academic research:
- Expansion of language capabilities to include non-English papers, expanding global research connections.
- Incorporation of adaptive features for users with various learning needs and preferences.
- Collaboration with educational institutions to make AI-generated summaries a standard tool in academic settings.